All Posts
Tue, 08 Oct 2024
What Are the Risks of Using AI in Banking?
Explore AI risks in banking, from data privacy to algorithmic bias, and discover strategies for safe, compliant AI adoption in financial institutions.
Artificial Intelligence (AI) has become a cornerstone in the banking industry, revolutionising processes ranging from customer service to fraud detection. However, while AI presents enormous benefits, it also introduces new risks that banks need to navigate carefully. Whether it’s data privacy concerns, algorithmic biases, or regulatory compliance, the adoption of AI in banking must be approached strategically.
In this article, we will explore the risks of using AI in banking, the potential challenges it presents, and what financial institutions can do to mitigate these risks. Our focus will remain on ensuring that banks can leverage AI safely and effectively while maintaining customer trust and adhering to regulatory standards.
Key Risks of Using AI in Banking
1. Data Privacy and Security Concerns
AI systems rely on vast amounts of data to function effectively. This data often includes sensitive financial and personal information, such as transaction histories, loan applications, and customer identities. If not properly secured, such data can become a prime target for cyberattacks.
- Challenge: As AI systems process and analyse large datasets, they become a potential vulnerability point for hackers. Breaches can lead to loss of sensitive information, resulting in legal repercussions and damage to the bank’s reputation.
- Mitigation Strategy: Implement robust encryption protocols and regularly update AI security measures. Use advanced data governance frameworks to ensure that all data used in AI models is protected and compliant with regulations like the General Data Protection Regulation (GDPR).
2. Algorithmic Bias and Discrimination
One of the most significant challenges with AI is ensuring that models are unbiased and equitable. AI algorithms learn from historical data, which can sometimes be skewed or biased against certain demographic groups. If left unchecked, these biases can lead to unfair lending practices, discriminatory decisions, and reputational harm.
- Challenge: Algorithms trained on biased datasets may favour certain customers over others, affecting credit decisions, loan approvals, and even fraud detection outcomes.
- Mitigation Strategy: Conduct regular audits of AI models to identify and correct biases. Use diverse training datasets and ensure that data inputs represent all demographics fairly. According to EY, banks should also implement “fairness constraints” in their algorithms to reduce potential discrimination.
3. Compliance and Regulatory Challenges
AI in banking must comply with a complex set of regulations that vary by jurisdiction. Regulations like the Bank Secrecy Act (BSA) and Anti-Money Laundering (AML) laws mandate that banks maintain transparency and accountability in their processes. Ensuring that AI systems adhere to these rules is crucial but can be challenging.
- Challenge: AI models are often “black boxes,” making it difficult to understand how they make decisions. This lack of transparency can complicate regulatory reporting and raise concerns with compliance officers.
- Mitigation Strategy: Implement explainable AI (XAI) techniques to make AI decision-making processes more transparent and interpretable. Use RegTech solutions to automate compliance and reporting, ensuring that AI models meet regulatory standards.
4. Over-Reliance on Automation
While AI can streamline operations, an over-reliance on automation can introduce new risks. If human oversight is reduced, the bank may miss critical red flags or fail to intervene in anomalous situations that require human judgement.
- Challenge: Automated systems may react too slowly to emerging risks or fail to consider nuanced situations that a human analyst would flag as suspicious.
- Mitigation Strategy: Maintain a balanced approach that combines AI with human oversight. Establish guidelines that define when a human should intervene in automated decision-making processes. According to ScienceDirect, banks should also establish “human-in-the-loop” systems to maintain control over critical decisions.
5. Model Drift and Performance Degradation
AI models can lose accuracy over time, a phenomenon known as “model drift.” This occurs when the underlying data or environment changes, causing the model to produce incorrect predictions. In the context of banking, model drift can lead to false positives in fraud detection or incorrect credit assessments.
- Challenge: Regularly updating models to reflect changing data patterns is resource-intensive but essential to maintain performance.
- Mitigation Strategy: Implement continuous monitoring systems to track model performance and detect drift early. Use automated retraining and testing processes to keep models up to date, as recommended by Ncontracts.
6. Lack of Transparency and Explainability
Many AI models, especially deep learning algorithms, are complex and difficult to interpret. This lack of explainability can pose a risk when banks need to justify their decisions to customers or regulators. Without clear explanations, stakeholders may lose trust in AI-driven outcomes.
- Challenge: Customers and regulators may not accept decisions that cannot be clearly explained or justified, even if the AI model is highly accurate.
- Mitigation Strategy: Implement Explainable AI (XAI) frameworks to provide understandable insights into how AI models make decisions. This approach not only builds trust but also simplifies compliance with transparency regulations like the California Consumer Privacy Act (CCPA).
Practical Solutions for Mitigating AI Risks in Banking
Given the risks associated with AI, banks must adopt a proactive approach to ensure that AI is implemented safely and responsibly. Here are some practical solutions to mitigate the key risks:
Data Governance: Establish a comprehensive data governance framework that covers data collection, storage, and use. Ensure compliance with regulations like GDPR and CCPA to protect customer data.
Bias Audits: Regularly audit AI models for biases and discriminatory outcomes. Use diverse datasets and incorporate fairness metrics to detect and correct biased patterns.
Human Oversight: Maintain a strong human oversight structure, especially for high-stakes decisions. Define clear protocols for when human intervention is necessary.
Model Validation and Monitoring: Use automated monitoring tools to detect model drift and performance degradation. Continuously validate models against new data to ensure accuracy and relevance.
Regulatory Compliance: Work closely with compliance officers to ensure that AI systems meet all regulatory requirements. Implement Explainable AI techniques to make decision-making processes more transparent.
Cybersecurity Measures: Invest in advanced cybersecurity solutions to protect AI systems from external threats. Regularly update and patch software to address vulnerabilities.
Fiskil: Enhancing Safe and Compliant AI Integration in Banking
What is Fiskil?
Fiskil is an open finance platform that provides secure and real-time access to banking and energy data. Built specifically for developers, Fiskil’s API infrastructure connects seamlessly to bank accounts, enabling real-time insights and robust data analysis. With its focus on compliance and security, Fiskil is an ideal partner for banks looking to implement AI responsibly.
How Fiskil Addresses AI Risks
Data Privacy and Security
Fiskil ensures that all data access is governed by stringent security protocols, complying with regulations like GDPR and the Consumer Data Right (CDR). This secure data access is crucial for AI models that rely on sensitive financial information.Compliance Management
Fiskil’s solutions are built with compliance in mind. The platform offers pre-built compliance modules, simplifying the process of adhering to regulations like AML and BSA.Real-Time Data Integration
With Fiskil’s real-time data integration, banks can maintain up-to-date AI models that reflect the latest transaction patterns. This reduces the risk of model drift and ensures ongoing accuracy.Explainable AI
Fiskil supports Explainable AI initiatives by providing transparent data access that makes it easier to interpret AI decisions. This transparency is critical for maintaining trust with customers and meeting regulatory requirements.
Why Choose Fiskil?
Fiskil’s powerful APIs provide secure, real-time access to banking data, enabling banks to build AI models that are not only effective but also safe and compliant. With Fiskil, financial institutions can reduce implementation risks, ensure transparency, and enhance customer trust. To learn more about how Fiskil can support your AI initiatives, visit the official Fiskil website or explore their blog for in-depth resources.
Conclusion
AI presents transformative opportunities for the banking sector, but it also introduces significant risks that must be carefully managed. From data privacy concerns to algorithmic biases, the adoption of AI requires a balanced approach that prioritises security, transparency, and compliance.
By implementing robust data governance, maintaining human oversight, and leveraging solutions like Fiskil, banks can harness the power of AI while mitigating potential risks. As AI continues to evolve, staying informed and adopting best practices will be essential for financial institutions to remain competitive and compliant in a rapidly changing environment.
Relevant Links
Fiskil Resources
Insights on AI for Fraud Detection in Banking
- Effectiv: Fraud Detection Using AI in Banking
- Infosys BPM: Fraud Detection with AI in the Banking Sector
- Hyperverge: AI for Fraud Prevention
- Instabase: AI in Fraud Detection for Banking
- LinkedIn: AI-Enabled Fraud Detection in Finance
- Deloitte: Deepfake Banking Fraud Risk on the Rise
- Docsumo: AI-Based Bank Fraud Detection
- GBG: AI's Role in Fighting Fraud in Financial Institutions
- SQN Banking Systems: AI in Bank Fraud Detection and Prevention
- Datategy: AI-Based Fraud Detection in Banks
Posted by
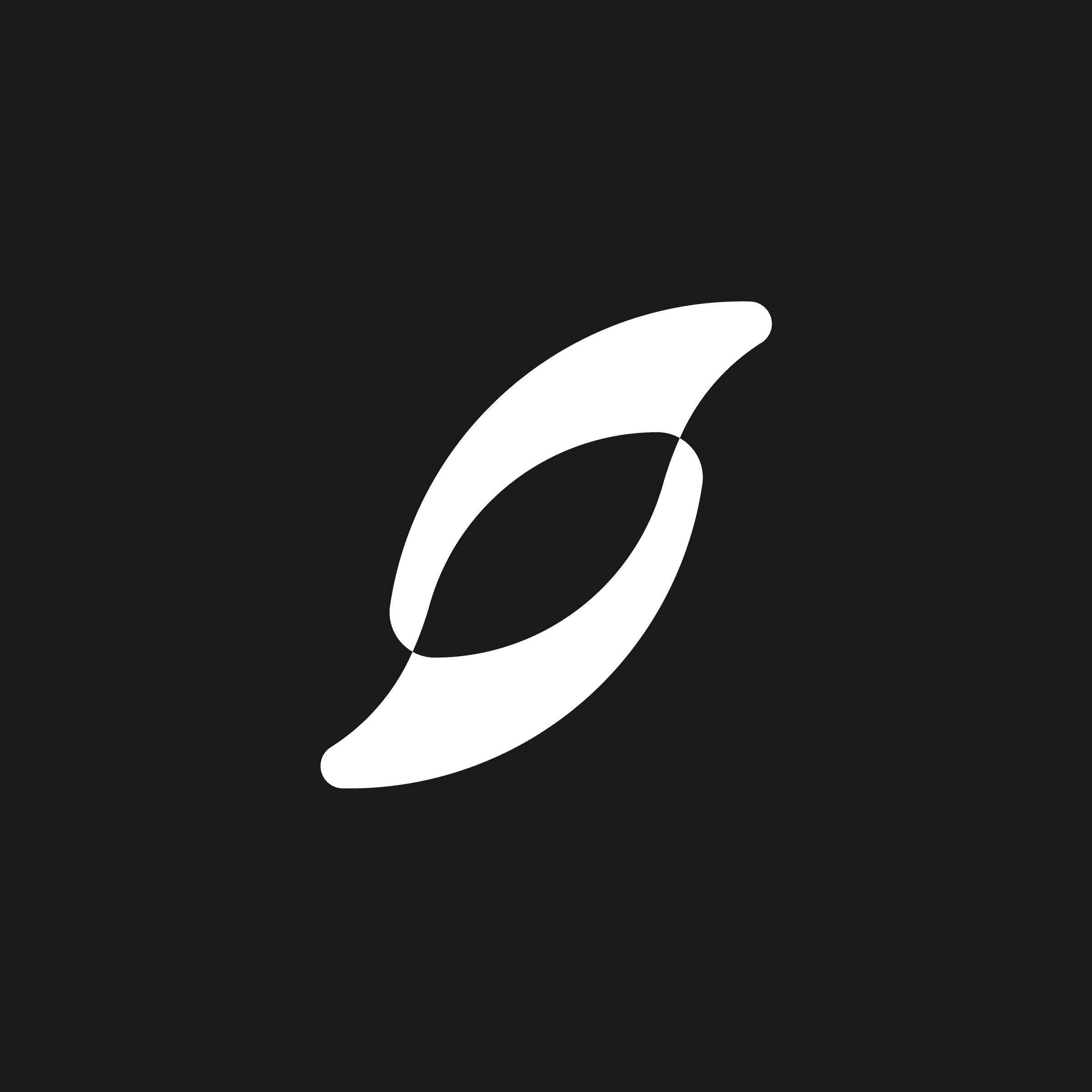
Fiskil
Share this post