All Posts
Tue, 08 Oct 2024
How Banks Are Utilizing AI for Fraud Detection
Discover how banks leverage AI for real-time fraud detection, enhancing security, compliance, and customer experience while reducing false positives.
As digital transactions continue to rise, so does the risk of fraud. To combat this growing threat, banks are increasingly turning to Artificial Intelligence (AI) to bolster their fraud detection systems. AI offers a robust solution, enabling financial institutions to monitor vast volumes of data in real time, identify suspicious patterns, and react to potential fraud faster than ever before. This technology not only protects banks and their customers but also ensures compliance with stringent financial regulations.
In this article, we will delve into how banks are using AI for fraud detection, the benefits it offers, and the challenges that come with integrating AI into traditional banking systems. We will also explore practical use cases and provide insights into how solutions like Fiskil are enhancing fraud detection capabilities.
What is AI Fraud Detection in Banking?
AI fraud detection in banking leverages advanced technologies such as machine learning, deep learning, and natural language processing to identify and prevent fraudulent activities. Unlike traditional methods, which rely on predefined rules, AI systems continuously learn and adapt to new fraud patterns by analysing large volumes of data in real-time. This dynamic approach allows financial institutions to detect even the most sophisticated fraud schemes and respond quickly, thereby safeguarding assets and maintaining customer trust (Effectiv).
How AI Prevents Fraud
AI enhances fraud detection through various methods, including:
- Behavioural Analysis: AI models monitor user behaviour patterns, such as login locations, transaction timings, and spending habits. Any deviation from the norm is flagged as potentially fraudulent.
- Anomaly Detection: AI detects unusual activities by comparing current transactions to historical data. For example, if a customer suddenly makes a high-value transaction in a foreign country, the system might flag it for review (Infosys BPM).
- Predictive Analytics: Machine learning models can forecast future fraudulent activities based on past data, helping banks stay ahead of emerging threats (Fraud.com).
- Link Analysis: By mapping relationships between different entities, AI can identify connections between fraudulent accounts, revealing organised fraud networks (F5).
AI-powered systems have been shown to reduce fraud losses by up to 50% for some major banks, highlighting their effectiveness in protecting both financial institutions and their customers (Effectiv).
How is Fraud Detection Currently Handled Without AI?
Traditional fraud detection methods rely heavily on rule-based systems and manual reviews. While these approaches have been the backbone of fraud prevention for years, they face significant limitations in today’s fast-paced, data-driven environment (Fraud.com).
Rule-Based Systems
Rule-based systems operate by using predefined rules and thresholds to flag transactions. For example, a rule might be set to alert the bank if a customer makes a transaction exceeding a specific amount or from an unusual location. While effective for simple fraud scenarios, these systems struggle to keep up with rapidly evolving fraud patterns.
- Limitations:
- High False Positives: Rule-based systems often produce a large number of false positives, which can lead to customer frustration and increased operational costs.
- Inflexibility: These systems lack the ability to adapt to new and sophisticated fraud tactics, making them less effective against complex fraud schemes (Infosys BPM).
- Reactive Approach: Traditional systems are more reactive than proactive, as they often identify fraud after it has occurred rather than preventing it in real-time (Effectiv).
Manual Reviews
In many cases, flagged transactions are sent to human analysts for manual review. While this approach ensures a high level of scrutiny, it is time-consuming, costly, and not scalable for high transaction volumes (F5).
- Challenges:
- Resource-Intensive: Manual reviews require a dedicated team of analysts, leading to increased labour costs and slower response times.
- Human Error: Analysts may overlook subtle patterns or make inconsistent decisions, leading to missed fraud cases.
- Scalability Issues: As the volume of digital transactions grows, manual reviews become increasingly impractical, creating bottlenecks in the fraud detection process (Fraud.com).
Static Data Analysis
Traditional systems often rely on static data points, such as transaction amounts and locations, without considering contextual information like customer behaviour over time. This makes it difficult to detect sophisticated fraud tactics that involve small, incremental changes (Infosys BPM).
- Example: A fraudster might gradually increase transaction amounts over time to evade detection by static threshold-based rules.
Given these limitations, traditional methods focus on “point-in-time” detection, flagging transactions that deviate from predefined norms. This approach often misses emerging fraud patterns that evolve over time, making it less effective against modern threats (Fraud.com).
Why Banks Need AI for Fraud Detection
Given the limitations of traditional methods, AI provides a much-needed upgrade to fraud detection capabilities. It enables real-time monitoring, reduces false positives, and offers a more proactive approach to managing fraud risk. As a result, AI has become a critical component of modern fraud detection strategies in the banking sector (Effectiv).
Why AI is Critical for Fraud Detection
Fraud detection has traditionally relied on rule-based systems that flag transactions based on predefined criteria. While effective to some extent, these systems often struggle to adapt to new and evolving fraud patterns. AI addresses these limitations through machine learning and deep learning algorithms that can:
- Identify Complex Patterns: AI can detect subtle correlations and non-linear relationships in data, which might be missed by traditional methods.
- Adapt and Learn: Machine learning models continuously learn from new data, improving their accuracy and ability to detect even the most sophisticated fraud schemes.
- Process Data at Scale: AI can analyse enormous datasets in real time, ensuring that fraudulent activities are detected and acted upon without delay.
According to Infosys, AI-powered systems have reduced fraud losses by up to 50% in some major financial institutions, demonstrating the technology’s effectiveness.
Key AI Techniques Used in Banking Fraud Detection
1. Machine Learning Algorithms
Machine learning (ML) models are central to AI-based fraud detection. These algorithms analyse historical transaction data to identify patterns associated with fraudulent activities. By training on large datasets, ML models can detect anomalies in real-time transactions, even if the suspicious behaviour has never been encountered before.
- Example: A bank using a supervised learning model to flag transactions where the user’s spending patterns suddenly change, indicating a potential account takeover.
2. Deep Learning and Neural Networks
Deep learning models, such as convolutional neural networks (CNN) and recurrent neural networks (RNN), are used to identify complex patterns in unstructured data, such as voice, video, and text. These models are highly effective in combating new types of fraud, including social engineering and deepfake scams.
- Use Case: An RNN model that monitors customer interactions with a bank’s virtual assistant, identifying voice patterns or phrases that are commonly used in phishing attempts.
3. Natural Language Processing (NLP)
NLP is used to analyse textual data, such as emails and chat messages, to detect phishing scams and other fraud attempts. By understanding the context and intent behind written communications, NLP can identify suspicious messages before they reach the customer.
- Example: An NLP-based system that scans incoming emails for fraudulent keywords, patterns, and social engineering tactics that are often employed to deceive customers.
4. Anomaly Detection
Anomaly detection is a technique where AI models analyse standard user behaviour and flag any deviations from the norm. This approach is particularly effective in detecting irregular spending patterns, unusual login locations, or sudden changes in transaction volumes.
- Use Case: A bank using anomaly detection to identify an account that suddenly starts making high-value transactions to foreign countries, which is inconsistent with the customer’s typical behaviour.
5. Graph Analysis
Graph-based AI models map relationships between different data points, such as user accounts, IP addresses, and devices, to identify suspicious connections that indicate fraudulent networks. Graph analysis helps banks detect organised fraud rings, which may not be apparent when viewing data in isolation.
- Use Case: A bank using graph analysis to detect a network of accounts that frequently transfer small amounts to each other before being consolidated into a single withdrawal.
Benefits of AI in Banking Fraud Detection
1. Real-Time Monitoring
AI systems enable banks to monitor transactions in real time, ensuring that suspicious activities are flagged and addressed immediately. According to Docsumo, this capability reduces the time to detect fraud from days to mere seconds, allowing for prompt action.
2. Reduced False Positives
Traditional fraud detection systems often generate high rates of false positives, leading to unnecessary investigations and customer dissatisfaction. AI’s advanced analytics can significantly reduce false positives, ensuring that only truly suspicious activities are flagged.
3. Enhanced Customer Experience
AI allows for more accurate and seamless fraud detection without disrupting legitimate transactions. This improves the overall customer experience, as genuine users are less likely to experience blocked transactions or verification delays.
4. Compliance with Regulations
With increasing regulatory scrutiny, banks are under pressure to ensure that their fraud detection systems comply with global standards like the Bank Secrecy Act (BSA) and Anti-Money Laundering (AML). AI systems can automate compliance checks and provide detailed audit trails, making regulatory adherence simpler and more effective.
Challenges of Implementing AI in Fraud Detection
Despite its benefits, implementing AI in banking fraud detection comes with several challenges:
Data Privacy Concerns: AI models require large volumes of data to function effectively, raising concerns about data privacy and security. Banks must ensure compliance with regulations like the General Data Protection Regulation (GDPR).
Integration with Legacy Systems: Many banks still rely on legacy systems that are not compatible with modern AI technologies. Integrating AI into these systems can be complex and costly.
Bias and Fairness: AI models can sometimes exhibit biases, especially if trained on non-representative datasets. Banks need to ensure that their AI models are transparent, fair, and do not inadvertently discriminate against certain customer groups.
Real-World Applications of AI in Fraud Detection
1. Credit Card Fraud Detection
Many banks use AI to monitor credit card transactions for signs of fraud. For example, if a card that is typically used in Australia is suddenly used in a different country, the AI system might flag it as suspicious and trigger an alert.
2. Anti-Money Laundering (AML) Compliance
AI is used to detect suspicious transaction patterns indicative of money laundering activities. Banks implement AI-based AML solutions to analyse transaction flows and identify unusual patterns that may indicate money laundering, such as frequent low-value transactions followed by a large cash withdrawal.
3. Account Takeover Detection
By analysing login patterns, IP addresses, and device data, AI can detect unusual login attempts and prevent account takeovers. This approach is particularly effective in stopping unauthorised access before it results in financial loss.
Introducing Fiskil: Enhancing Fraud Detection with AI-Driven Solutions
What is Fiskil?
Fiskil is a cutting-edge open finance platform that provides seamless access to real-time banking and energy data. Built specifically for developers, Fiskil’s API infrastructure connects directly to users’ bank accounts, allowing for deep insights and robust data analytics that enhance AI capabilities in fraud detection and compliance.
How Fiskil Elevates AI-Based Fraud Detection
Real-Time Data Access
Fiskil’s APIs provide real-time access to bank transactions, enabling AI models to detect anomalies and suspicious activities as they happen. This real-time capability is crucial for stopping fraud before it escalates.Identity Verification
With direct access to users’ bank data, Fiskil simplifies identity verification, ensuring that transactions are conducted by the rightful account holders. This reduces the risk of identity theft and account takeovers.Enhanced Compliance Management
Fiskil offers pre-built compliance solutions, making it easier for banks to adhere to regulations like AML and KYC. This ensures that fraud detection models are not only effective but also compliant with legal standards.Scalable Infrastructure
Fiskil’s infrastructure supports large-scale AI deployments, making it ideal for banks looking to enhance their fraud detection capabilities across multiple markets and regions.
Why Choose Fiskil?
Fiskil’s robust APIs connect directly to users’ bank accounts, providing real-time data access that fuels AI-driven fraud detection. With Fiskil, banks can build more reliable and efficient fraud detection systems, reduce false positives, and maintain compliance effortlessly. To learn more about Fiskil’s solutions, visit the official Fiskil website or explore their blog for additional resources.
Conclusion
AI is revolutionising fraud detection in banking, providing faster, more accurate, and scalable solutions to combat the ever-evolving landscape of financial crime. By utilising AI, banks can protect their customers, maintain compliance, and ensure operational efficiency. While challenges remain, the potential benefits of AI far outweigh the risks.
With solutions like Fiskil, banks can elevate their AI-driven fraud detection systems, ensuring that they are not only effective but also compliant and future-ready. The integration of real-time data, automated compliance, and scalable infrastructure positions Fiskil as a valuable partner for banks aiming to enhance their fraud detection capabilities.
The future of banking fraud detection is here, and it’s powered by AI.
Relevant Links
Fiskil Resources
Insights on AI for Fraud Detection in Banking
- Effectiv: Fraud Detection Using AI in Banking
- Infosys BPM: Fraud Detection with AI in the Banking Sector
- Hyperverge: AI for Fraud Prevention
- Instabase: AI in Fraud Detection for Banking
- LinkedIn: AI-Enabled Fraud Detection in Finance
- Deloitte: Deepfake Banking Fraud Risk on the Rise
- Docsumo: AI-Based Bank Fraud Detection
- GBG: AI's Role in Fighting Fraud in Financial Institutions
- SQN Banking Systems: AI in Bank Fraud Detection and Prevention
- Datategy: AI-Based Fraud Detection in Banks
Posted by
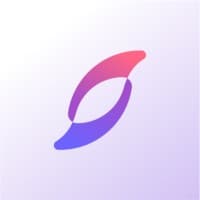
Fiskil
Share this post